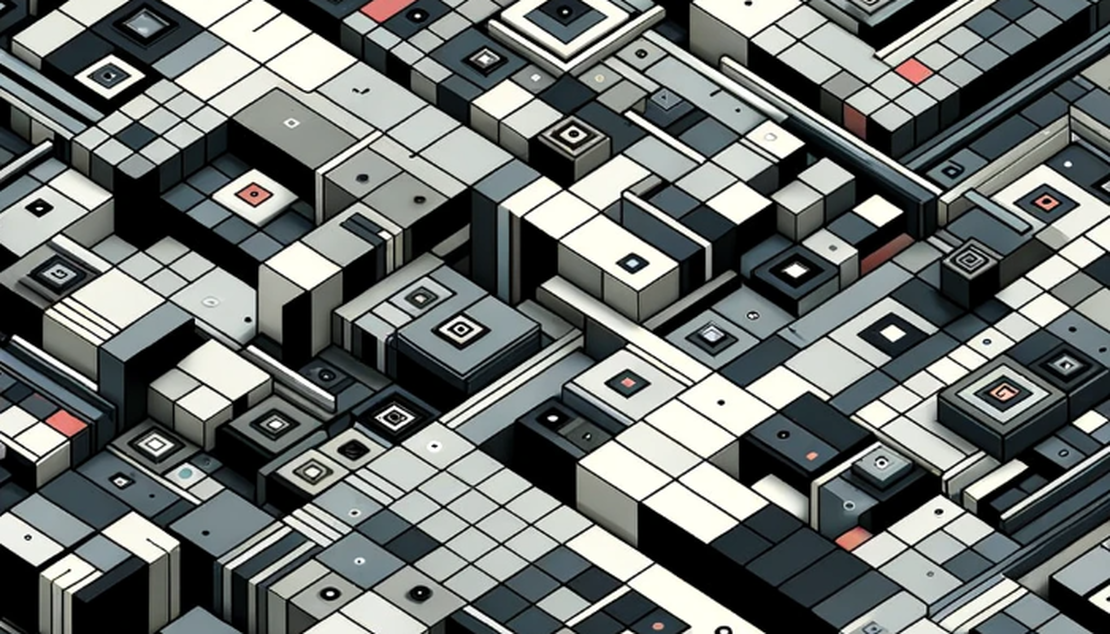
LLMs for SaaS: Beyond the Hype
- Cognesy Team
- Applications
- December 5, 2023
Large Language Models. They are all over news and social media - do they have any practical applications?
If you run a SaaS business, you cannot (unlike marketers or Twitter influencers) just chase the next big thing; you’re looking for tools that genuinely make work easier and more efficient.
So, how can this new tech actually help your business? Let’s talk about it.
Marketing: Content Generation and Analysis
Obvious application of LLMs is content authoring. You can use LLM based tools to draft Twitter posts or blog content based on some existing materials, saving you time. LLMs can also process competitor web content, helping identify gaps in your marketing strategy. Custom solutions using vector databases (like Pinecone or Weaviate) can assist analyzing semantic trends to better understand how your competitors’ positioning is changing and to keep your content relevant and timely.
Use cases
- Content Automation - Automated drafting of content (such as social media posts, blog articles, FAQs, and website updates, all based on your existing company materials.
- Competitive Analysis - Automated extraction and summarization of competitor information and news, providing a foundation for competitive strategies. Finding differences and gaps in features or messaging compared to competitors, aiding in refining your marketing approach and materials.
- Responding to Market Changes - Identifying opportunities with automated content summarization and comprehension - market developments like acquisitions or regulatory changes, to save time or respond fast.
Operations: Better Customer Support
Operational tasks like responding to customer inquiries and sorting support tickets can be efficiently managed with LLMs. Language models can understand and help respond to various customer queries, improving response time and reducing effort.
Use cases
- Responding to Customer Inquiries: Handling customer questions, e.g. about billing or products. LLM based solution can be turned into an interactive knowledge base with a chatbot answering or guiding user. This can augment product documentation and lead to better customer experience.
- Automating Support Tickets: Generating automated responses (or drafts) to certain types of support tickets (typically ’low-stakes’ ones).
- Personalized Customer Messaging: Creating personalized customer lifecycle messages, based on their activities to help with activation and engagement.
Sales: Conversational AI for Customer Engagement Conversational
AI can increase sales - chatbots embedded on websites can initiate and drive conversations with site viewers, answering their questions and guiding them through the sales funnel, 24/7. They can follow rules described in a natural language, asking questions to qualify lead for your product or service and addressing objections.
Use cases
- Visitor to Customer Conversion: Chatbots can initiate and sustain natural-feeling conversations with site visitors, converting website visitors into customers while answering visitors questions on your product, service or pricing.
- Tailored Conversations: Chatbots can feel natural, yet follow specified script, asking questions to assess the suitability of customers for your product, addressing objections and building familiarity with your offering.
- Better Insight: The ability to monitor chatbot conversations and improve interactions based on recorded chats, for example by storing “golden answers” in vector DB and prioritizing them for answer generation.
Product Development: New Tool for Feature Request Analysis
LLMs can help you automate the process of parsing new product issue reports or feature requests. LLM can comprehend the text, differentiate between new ideas and existing features, or assess the urgency of user needs. Couple this with a vector database, and you can cluster these requests for more organized review.
Use cases
- Duplication Detection: Identifying whether a request is a repeat or mirrors an existing feature, thereby avoiding redundancy.
- Rapid Assessment of Requests: Automated matching of feature request with your product’s capabilities and roadmap.
- Emotional Temperature Check: Assessing the tone of user communications to understand sentiment or urgency. Can be used to evaluating whether a request is a minor suggestion, a moderate inconvenience, or a critical pain point for users.
Customer Retention and Referrals: Sentiment Analysis and Advocacy
Identifying customer sentiment is crucial for retention. LLMs can analyse communication content to highlight potential retention risks. For referrals, these technologies can pinpoint and utilize positive feedback, helping to turn them into testimonials.
Use cases
- Customer Sentiment Analysis: Analyze customer feelings from surveys or their communications (e.g. support emails or messages), pinpointing those at risk of leaving.
- Automated Testimonial Drafting: Automatically extracting and drafting testimonials from positive statements in emails, support tickets, or forums.
- Scaling Customer Advocacy: Creating ready-to-use advocacy content, ideal for businesses with many small to medium-sized customers.
LLMs are not just a fancy trend; it’s a new tool that can help solve complex tasks, which previously required tons of effort and could not scale well.
What do you think? How do you use Large Language Models in your business? Feel free to comment below.